Artificial Intelligence (AI) has emerged as a transformative force in the cybersecurity landscape, redefining how organizations and individuals protect their digital assets. In an era where cyber threats are growing more sophisticated and pervasive, AI provides the tools and methodologies necessary to anticipate, identify, and neutralize these risks. This blog delves into the multifaceted role of AI in modern cybersecurity, exploring its benefits, challenges, and the future it heralds for digital defense.
Understanding the Intersection of Artificial Intelligence in Modern Cybersecurity
The evolution of AI has brought about unprecedented advancements in data processing, pattern recognition, and predictive analytics. These capabilities are particularly valuable in cybersecurity, where detecting anomalies and rapid threat responses are crucial. Traditional cybersecurity methods often rely on static rules and signature-based detection, but as cybercriminals adapt, these methods struggle to keep pace. AI introduces dynamic, learning-based approaches that can continuously adapt to new and evolving threats.
AI-powered systems analyze vast amounts of data at high speeds, identifying patterns that may indicate malicious behavior. By leveraging machine learning (ML) and deep learning algorithms, cybersecurity solutions can uncover hidden threats that might otherwise evade detection. This transformation from reactive to proactive security is one of the key contributions of AI in the modern threat landscape.
How AI Enhances Threat Detection
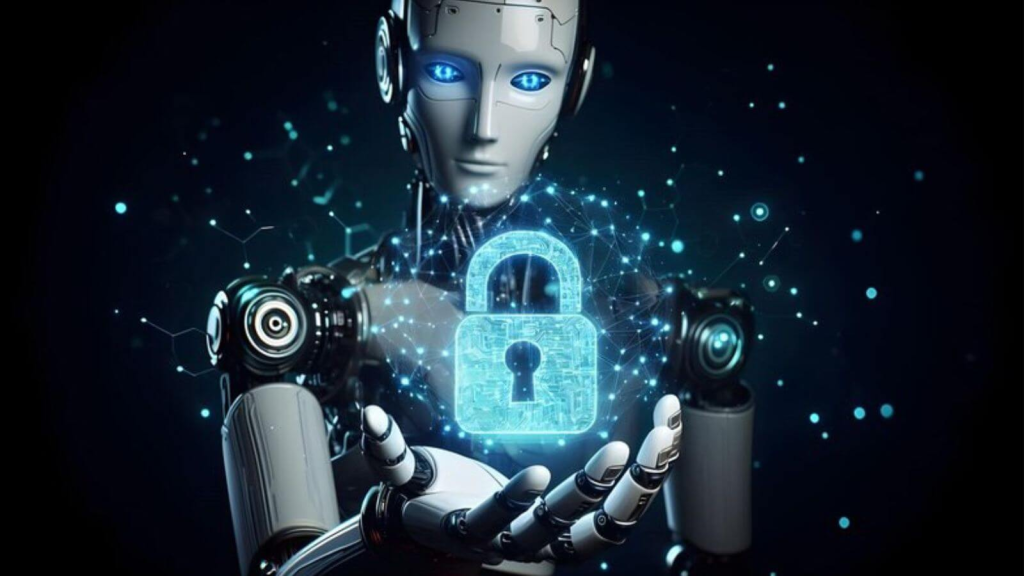
AI’s ability to process and analyze large datasets in real-time makes it an indispensable tool for threat detection. Machine learning models are trained on historical data to recognize patterns and indicators of compromise, which then help in predicting future attacks. As new data flows in, these models update their algorithms, learning from both successes and failures. This continuous learning cycle is a game-changer in combating advanced persistent threats (APTs) and zero-day exploits.
Real-Time Anomaly Detection
One of the most significant contributions of AI in cybersecurity is its capability for real-time anomaly detection. Traditional systems often fail when a threat deviates from a known pattern. AI, on the other hand, uses anomaly detection algorithms to flag deviations in network traffic, system behavior, and user activities. This proactive approach allows organizations to detect and respond to threats much faster than ever before.
Behavioral Analysis and Pattern Recognition
AI systems excel in behavioral analysis, monitoring user and entity behavior over time to establish a baseline of normal activity. When deviations occur—such as unusual login times, atypical data access, or abnormal transaction patterns—the system raises alerts. This behavioral insight is essential in detecting insider threats and compromised accounts, where the malicious activity might be subtle and difficult to identify using conventional methods.
Threat Intelligence and Predictive Analytics
By integrating threat intelligence feeds with AI-powered predictive analytics, cybersecurity solutions can forecast potential attack vectors. This synthesis of external intelligence and internal data enables organizations to prepare for and mitigate risks before they escalate. Predictive models can identify emerging trends and vulnerabilities, offering a strategic advantage by enabling preemptive action against likely threats.
Advancements in Machine Learning and Deep Learning Applications
Machine learning and deep learning are the cornerstones of AI-driven cybersecurity. These technologies allow systems to learn from experience, adapt to new threats, and improve their decision-making processes over time.
Supervised and Unsupervised Learning in Cybersecurity
In supervised learning, AI models are trained on labeled datasets where each data point is tagged with the correct outcome. This approach is highly effective for known threats, enabling the model to classify and react to similar patterns in real time. Conversely, unsupervised learning does not rely on labeled data, making it invaluable for discovering unknown threats or new variants of malware. By analyzing raw data, unsupervised models can cluster behaviors and flag anomalies without predefined rules.
Deep Learning for Complex Threat Landscapes
Deep learning, a subset of machine learning, employs neural networks with multiple layers to process complex patterns in data. These networks excel in recognizing intricate patterns and correlations that are not immediately apparent. In cybersecurity, deep learning algorithms are used for tasks such as image recognition in phishing detection, natural language processing in monitoring communications, and even voice recognition in secure access systems. The ability of deep learning to handle unstructured data sets it apart, providing a robust solution for modern cybersecurity challenges.
AI in Automated Incident Response
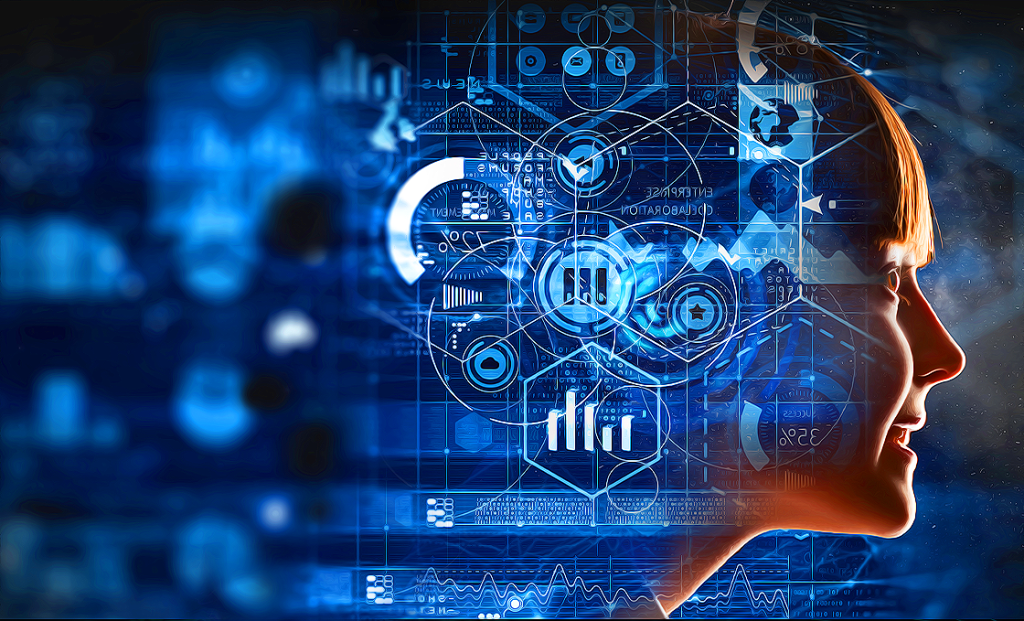
The rapid pace of cyberattacks requires immediate responses to minimize damage. AI-driven automation in incident response is a vital development that reduces the time between detection and remediation. Automated systems can contain breaches, isolate affected systems, and initiate recovery protocols without waiting for human intervention.
Automated Threat Containment
When an AI system detects a potential threat, it can automatically isolate affected endpoints or network segments. This containment strategy is crucial in preventing the lateral spread of malware and other forms of cyber intrusions. By acting swiftly, automated incident response systems help mitigate the impact of an attack, reducing downtime and limiting data loss.
Enhancing Human Decision-Making
While automation plays a critical role, AI also augments the capabilities of cybersecurity professionals. By filtering out false positives and providing actionable insights, AI empowers security teams to make informed decisions quickly. This collaboration between human expertise and machine efficiency creates a more resilient cybersecurity infrastructure where real-time data and automated processes support strategic decision-making.
The Integration of AI with Traditional Cybersecurity Frameworks
AI does not replace traditional cybersecurity methods; rather, it enhances them. The integration of AI into existing cybersecurity frameworks creates a layered defense strategy that is both robust and adaptive.
Augmenting Signature-Based Systems
Signature-based systems have long been the backbone of cybersecurity, relying on known patterns to detect threats. AI complements these systems by identifying threats that do not match any known signatures. This hybrid approach ensures that both known and unknown threats are addressed, significantly reducing the risk of a successful attack.
Enhancing Endpoint Security
Endpoints—such as laptops, mobile devices, and IoT devices—are often the weakest links in cybersecurity. AI-driven endpoint protection solutions monitor these devices continuously, identifying suspicious activities and potential vulnerabilities. By integrating AI with traditional antivirus and firewall systems, organizations can achieve a more comprehensive defense that covers all potential points of entry.
Overcoming Challenges in AI-Driven Cybersecurity
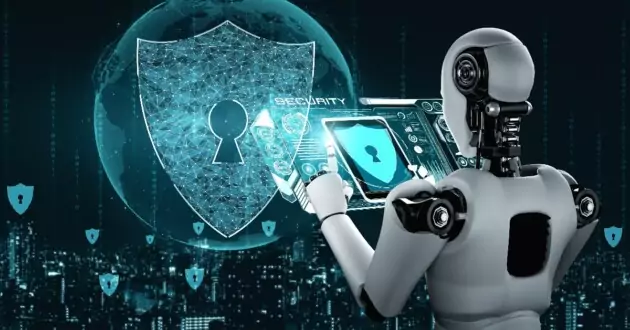
Despite its many benefits, the implementation of AI in cybersecurity is not without challenges. Issues related to data privacy, algorithmic bias, and the potential for adversarial attacks are at the forefront of ongoing research and development.
Data Privacy and Ethical Considerations
AI systems require vast amounts of data to function effectively. However, this data often includes sensitive information, raising concerns about privacy and compliance with data protection regulations. Organizations must balance the benefits of AI-driven analysis with the need to protect individual privacy and adhere to legal requirements. Transparent data governance policies and robust anonymization techniques are essential to mitigate these concerns.
Algorithmic Bias and False Positives
One of the risks of AI is the potential for algorithmic bias, where skewed or unrepresentative data influence the system’s learning. This bias can lead to false positives or even the misclassification of legitimate activities as malicious. Continuous monitoring, regular updates, and the inclusion of diverse datasets are critical to ensure that AI systems remain fair and effective in detecting threats.
Adversarial Attacks on AI Systems
Cyber adversaries are constantly evolving their tactics, and AI systems are not immune to these threats. Adversarial attacks involve manipulating input data to deceive AI models, causing them to misclassify or overlook malicious activities. Researchers are actively developing strategies to harden AI models against such attacks, including adversarial training and improved anomaly detection algorithms. It is a constant cat-and-mouse game, with each side striving to outmaneuver the other.
Case Studies and Real-world Applications
Real-world implementations of AI in cybersecurity highlight its potential and the tangible benefits it brings to organizations across various sectors. From financial institutions to healthcare providers, AI-driven solutions are being deployed to combat sophisticated cyber threats.
Financial Sector and Fraud Detection
Financial institutions are prime targets for cybercriminals due to the valuable data and financial resources they possess. AI is used extensively in fraud detection, where it analyzes transaction patterns and customer behavior to flag suspicious activities. These systems not only detect fraud in real-time but also help in predicting potential fraudulent schemes based on historical data. As cyber threats in the financial sector become more complex, AI continues to be an indispensable tool in maintaining the integrity and trust of financial systems.
Healthcare and Protecting Sensitive Data
The healthcare industry is another critical area where cybersecurity is paramount. With the increasing digitization of medical records and the integration of IoT devices in patient care, healthcare providers face significant cyber threats. AI is deployed to safeguard patient data, detect unauthorized access, and ensure the continuous availability of critical healthcare services. By automating threat detection and response, AI helps healthcare organizations maintain compliance with stringent data protection regulations while protecting sensitive patient information.
Government and National Security
Government agencies and national security organizations are at the forefront of adopting AI-driven cybersecurity solutions. These entities are tasked with protecting national infrastructure, sensitive government communications, and classified data from cyber espionage and state-sponsored attacks. AI systems are used to monitor network traffic, detect anomalies, and respond to threats at a scale that would be impossible with traditional methods alone. The integration of AI in national security underscores its strategic importance in defending against cyber warfare and maintaining public trust.
The Future of AI in Cybersecurity
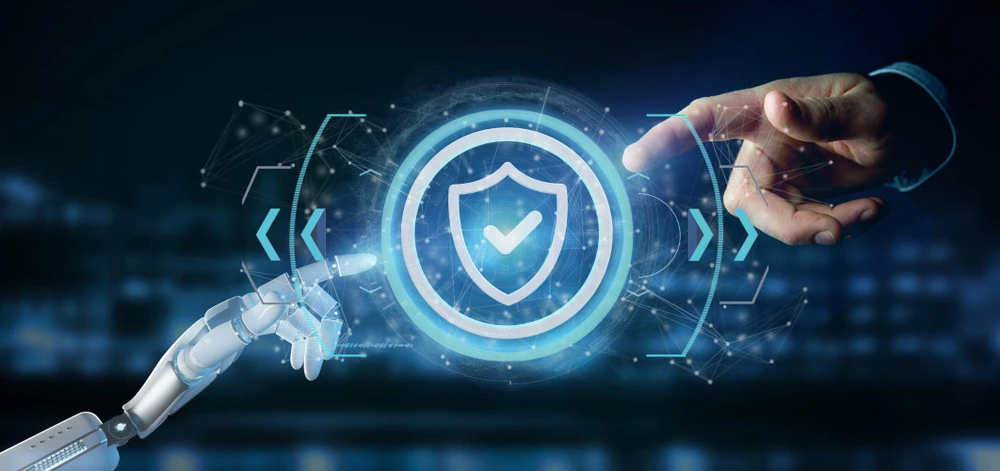
The future of AI in cybersecurity is both promising and challenging. As AI technologies continue to evolve, they will bring even greater capabilities to the field, from enhanced threat prediction to more sophisticated automated responses. However, the rapid pace of technological advancement also means that cybercriminals will continue to develop new methods to exploit vulnerabilities in AI systems.
Continuous Learning and Adaptation
One of the most exciting prospects for the future is the development of AI systems that can continuously learn and adapt without human intervention. These systems will be able to evolve alongside cyber threats, automatically updating their algorithms and strategies based on real-time data. The concept of self-healing networks—where systems detect and repair vulnerabilities autonomously—is gaining traction and could revolutionize how cybersecurity is managed in the coming years.
Collaborative Defense Mechanisms
As AI becomes more integral to cybersecurity, collaboration between organizations, governments, and research institutions will be key. Sharing threat intelligence, best practices and innovations in AI technology will help create a unified defense mechanism capable of responding to cyber threats on a global scale. Public-private partnerships and international collaborations are likely to play a significant role in shaping the future landscape of AI-driven cybersecurity.
Balancing Innovation with Regulation
While the benefits of AI in cybersecurity are immense, there is also a growing need for regulation and standardization. As organizations increasingly rely on AI for critical security functions, ensuring that these systems are secure, transparent, and ethical becomes paramount. Regulatory frameworks will need to evolve to address the unique challenges posed by AI, balancing innovation with the need to protect data privacy and prevent misuse. The future will likely see the emergence of international standards that guide the ethical and secure deployment of AI in cybersecurity.
Conclusion
Artificial Intelligence is not just a technological innovation—it is a paradigm shift in how cybersecurity is approached and managed. By harnessing the power of AI, organizations can transition from reactive to proactive security measures, effectively anticipating and mitigating threats before they cause harm. From real-time anomaly detection to automated incident response and predictive analytics, AI is reshaping the cybersecurity landscape in profound ways.
The integration of AI with traditional cybersecurity frameworks creates a layered, dynamic defense system that is both robust and adaptable. As cyber threats continue to evolve, the role of AI will only become more critical. While challenges such as data privacy, algorithmic bias, and adversarial attacks remain, ongoing research and collaboration are paving the way for more resilient and secure AI systems.